
A Deeper Insight on How the Ecosystem Concept may Affect the Implementation of AI in Pathology
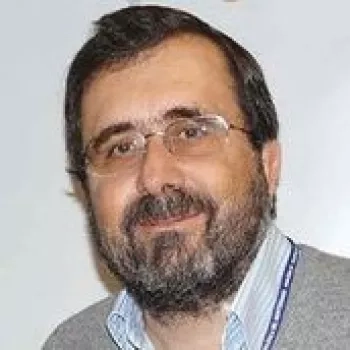
An integrated digital pathology ecosystem is only possible to maintain in the long term when international interoperability standards are followed. It must be envisioned as a global strategy comprising not only imaging data.
Digital pathology provides for better patient healthcare by offering excellent quality in pathology diagnosis, improving efficiency in microscopic evaluation, minimizing eventual errors (e.g., making sure that the whole slide has been reviewed), and allowing for automation of image analysis, to improve objectivity and reproducibility in routine tasks like biomarker quantification.
In the evolution towards personalized medicine, the Systems Pathology concept was established by 2010, and it was defined as the integration of clinical, morphological, molecular, and quantitative assessment using new mathematical methodologies 1. Other approaches, like Computational Pathology, are also based on an integrated view of medical informatics and diagnostic machine learning 2.
The integration of AI in pathology must be envisioned as a global strategy, comprising not only imaging data. New natural language processing algorithms play a very interesting role in voice recognition, automatic coding, synoptic reports completion, or data analytics, amongst others. But deep learning also offers a new approach in Next-Generation Sequencing (NGS) data processing, since convolutional neural networks are being trained to improve NGS variant filtering and the rest of the nucleic acid sequencing workflow 3.
Furthermore, this global strategy should embrace not only pathology but also radiology and -omics data in a big data convergence approach, as described by Madabhushi and Lee 4.
This integrated digital pathology ecosystem is only possible to maintain in the long term when international interoperability standards are followed. An effective integration of AI solutions in digital pathology can also benefit from standards and initiatives like Health Level Seven (HL7), Fast Healthcare Interoperability Resources (FHIR) for messaging between information systems, Digital Imaging and Communications in Medicine (DICOM) for image management, and Systematized Nomenclature of Medicine – Clinical Terms (SNOMED CT) for coding and concept normalization. Integrating the Healthcare Enterprise (IHE) defines how to use all these standards, which also includes a Pathology and Laboratory Medicine (PaLM) domain.
Whole slide imaging (WSI) scanner manufacturers are also helping in this evolution of digital pathology towards artificial intelligence, by implementing the capability to directly generate DICOM files, accepting HL7 messaging, or adapting other international standards, like ICC profiles in color management.
We also must be prepared for the integration of emerging technologies (multiphoton microscopy, spatially resolved transcriptomics). Some of these technologies may significantly affect first research workflow, and later, clinical workflow. For instance, using deep learning algorithms applied to multiphoton microscopy, it is possible to distinguish cancerous versus non-cancerous tissue with 100% accuracy, and this approach has been suggested for rapid intra-operative assessment of glioma surgery 5. One of the advantages of deep learning in AI is the capability to work simultaneously with multiple types of data from different sources to discover new predictions and link different data together. In this sense, hematoxylin-eosin digital slides can be used by a deep learning algorithm to predict the immunohistochemical expression, the possible geographical probability of DNA or RNA mutations in the tissue, the specific treatment response, or even the overall survival of a patient.
There are very interesting approaches for designing Content-Based Image Retrieval (CBIR) systems based on deep learning to implement efficient search engines in pathology 6. Other authors have connected hematoxylin-eosin with genomics by defining a dictionary of “visual words” derived from images that allow linking phenotypical features extracted from images with some molecular pathway activity to predict prognosis in low grade gliomas 7.
Deep learning algorithms can extract an unforeseen amount of data from hematoxylin-eosin or Papanicolaou stained sections, finding new morphological patterns or helping in objective quantification. This will allow an even greater boost in the use of these basic stains, being such affordable techniques and their high cost-effectiveness in diagnosis.
발표자 소개
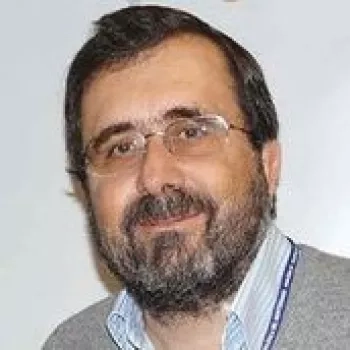
Dr. García-Rojo is Head of the Pathology Department at Hospital Universitario Puerta del Mar, in Cádiz, Spain.
He is the Past-President of the European Society of Digital and Integrative Pathology, and past-President of the Ibero-American Association for Telemedicine and Telehealth (IATT).
Dr. García-Rojo Is the former chair of the European project EURO-TELEPATH, Anatomic Telepathology Network, Action IC0604 of the European Cooperation in the field of Scientific and Technical Research (COST).
He received his PhD from Universidad Autónoma, Madrid. Dr. García-Rojo’s main research areas are medical informatics standards in digital pathology and molecular pathology. He has published three books on medical informatics, five electronic publications (CD/DVD) and is the author of 23 book chapters and 125 scientific journal papers.
참조 문헌
- Faratian D, Clyde R, Crawford J, et al. Systems pathology—taking molecular pathology into a new dimension. Nat Rev Clin Oncol. 2009; 6: 455–464. Available at: https://www.nature.com/articles/nrclinonc.2009.102
- Fuchs TJ, Buhmann JM. Computational pathology: challenges and promises for tissue analysis. Comput Med Imaging Graph. 2011; 35(7-8): 515-530. Available at: https://www.sciencedirect.com/science/article/abs/pii/S0895611111000383
- Celesti F, Celesti A, Wan J, Villari M. Why Deep Learning Is Changing the Way to Approach NGS Data Processing: A Review. IEEE Rev Biomed Eng. 2018; 11: 68-76. Available at: https://ieeexplore.ieee.org/document/8336918
- Madabhushi A, Lee G. Image analysis and machine learning in digital pathology: Challenges and opportunities. Med Image Anal. 2016; 33: 170-175. Available at: https://www.sciencedirect.com/science/article/pii/S1361841516301141
- Chen D, Nauen DW, Park HC, Li D, Yuan W, Li A, Guan H, Kut C, Chaichana KL, Bettegowda C, Quiñones-Hinojosa A, Li X. Label-free imaging of human brain tissue at subcellular resolution for potential rapid intra-operative assessment of glioma surgery. Theranostics. 2021; 11(15): 7222-7234. Available at: https://www.ncbi.nlm.nih.gov/pmc/articles/PMC8210590/
- Schaer R, Otálora S, Jimenez-Del-Toro O, Atzori M, Müller H. Deep Learning-Based Retrieval System for Gigapixel Histopathology Cases and the Open Access Literature. J Pathol Inform. 2019 Jul 1;10:19. Available at: https://www.ncbi.nlm.nih.gov/pmc/articles/PMC6639847/
- Powell RT, et al. Identification of Histological Correlates of Overall Survival in Lower Grade Gliomas Using a Bag-of-words Paradigm: A Preliminary Analysis Based on Hematoxylin & Eosin Stained Slides from the Lower Grade Glioma Cohort of The Cancer Genome Atlas. J Pathol Inform. 2017; 8: 9. https://www.jpathinformatics.org/text.asp?2017/8/1/9/201916.
Related Content
라이카 바이오시스템즈 Knowledge Pathway 콘텐츠는 에서 이용할 수 있는 라이카 바이오시스템즈 웹사이트 이용 약관의 적용을 받습니다. 법적고지. 라이카 바이오시스템즈 웨비나, 교육 프레젠테이션 및 관련 자료는 특별 주제 관련 일반 정보를 제공하지만 의료, 규정 또는 법률 상담으로 제공되지 않으며 해석되어서는 안 됩니다. 관점과 의견은 발표자/저자의 개인 관점과 의견이며 라이카 바이오시스템즈, 그 직원 또는 대행사의 관점이나 의견을 나타내거나 반영하지 않습니다. 제3자 자원 또는 콘텐츠에 대한 액세스를 제공하는 콘텐츠에 포함된 모든 링크는 오직 편의를 위해 제공됩니다.
모든 제품 사용에 다양한 제품 및 장치의 제품 정보 가이드, 부속 문서 및 작동 설명서를 참조해야 합니다.
Copyright © 2025 Leica Biosystems division of Leica Microsystems, Inc. and its Leica Biosystems affiliates. All rights reserved. LEICA and the Leica Logo are registered trademarks of Leica Microsystems IR GmbH.